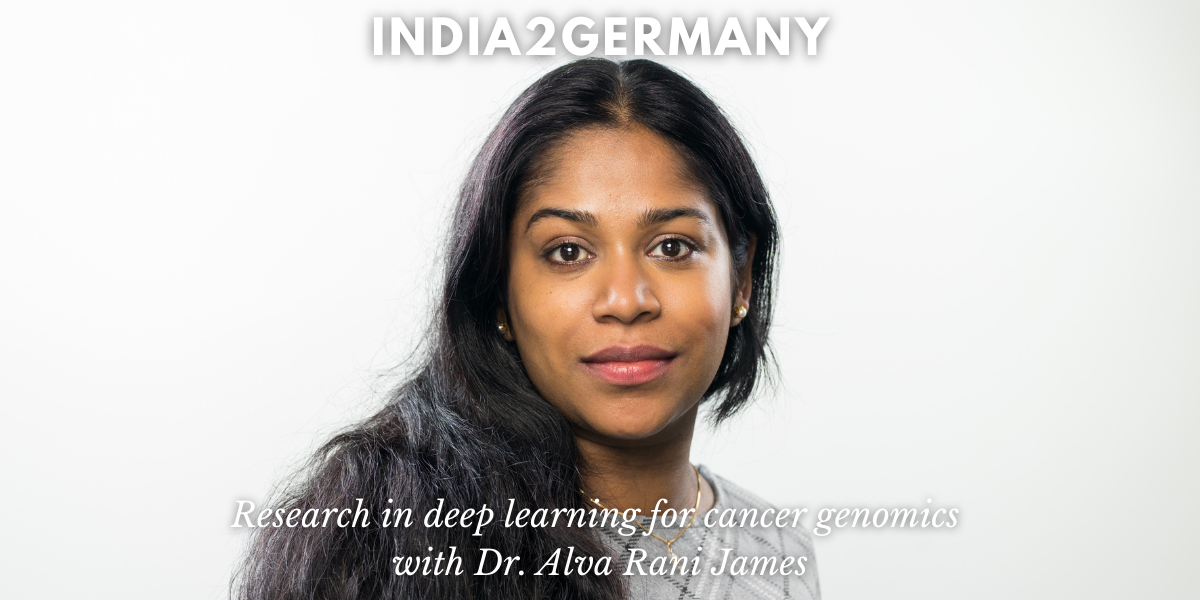
Research in deep learning for cancer genomics with Dr. Alva Rani James
Alva gives an insight into the topic of using deep learning on genomic data to support clinicians in decision making for cancer patients. She also shares her advice for people looking to work in the field of cancer genomics.
Understanding the genomic changes associated with various types of cancer has perhaps been one of the most important topics of research in the past decade. Today we speak with Dr. Alva Rani James - a bionformatician working as Postdoc at the Hasso Plattner Institute in Potsdam, Germany who is using deep learning on genomic sequences to delve deeper into cancer genomics. Alva's research career spans highly prestigious institutions, such as the Charité University Hospital, German Cancer Research Center, ETH Zurich and Karolinska Institute. She is highly experienced in building bioinformatic and statistical pipelines for analyzing human big data. Alva shares her learnings and advice for those looking to build a career in analysing human big data using machine learning.
🎙 Subscribe to the India2Germany podcast on Spotify, Apple Podcasts, Google Podcasts, YouTube or any other platform to be the first to know when a new episode is released 🙂
Hi Alva 👋 What exactly does your work focus on?
My work focuses on elucidating the functions of cancer related RNAs or genes, involved in certain signaling pathways. The signaling pathways are the pathways that give signals to the cancer cells to grow, proliferate and spread across the body, and also to elucidate those genes and their tumor-suppressing activity across multiple cancer types. Our focus is to look mainly into a particular kind of genes, known as non-coding genes. Those are already reported to be involved in multiple hallmarks of cancer including progression, metastasis, proliferation and so on. So the goal at the end would be to develop a tool that would help physicians to stratify the patients based on their risk group and plan the treatment protocol. Technically, it is like a system that supports Clinical Decision Making by leveraging latest developments in deep learning and bioinformatics, especially in combination. Besides this I am also involved in developing multiple reproducible workflows or pipelines for various projects; we will publish it for the community, so that people can use it free of cost.
Cancer is a heterogeneous disease, meaning that it is not a single disease. It is a group of diseases having multiple subtypes. For instance, leukemia (blood cancer) has multiple subtypes, like Philadelphia positive, Philadelphia negative, so on and so forth. Each subgroup requires specific treatment.
Some subgroups need less aggressive treatment protocol while another needs more aggressive treatment like chemotherapy. Hence, it is necessary that the clinician gets to know what subgroup or which group the patient belongs to, so that he can determine what type of treatment protocol he shall follow for each patient. Thus the life quality of the patient and the palliative care for the patient will improve drastically. That is the goal at the end.
Do you focus on a specific form of cancer?
Currently we are exploring to find out the functions of a particular kind of RNAs, that I mentioned earlier, across multiple cancer types because we have consortia where the tumor samples are available for more than 20 tumor types and their subtypes. There are around 250 plus subtypes available from thousands of patients. It is a consortium from the US, from the National Institute of Health called The Cancer Genome Atlas, abbreviated as TCGA, were you can use the public data and work around with multiple cancers. But then eventually to translate this model to our hospital, we really need to have it validated and for which we can only start with a single cancer. It is based on the availability of samples from the hospital. In the beginning we will start with multiple cancers and later narrow it down. That is the plan, but then it is a long term goal.
Anyone suspecting of having cancer goes to the hospital, where for the diagnosis a blood sample is taken. From the blood, the DNA and RNA molecules are extracted, which is pretty stable, later it goes into a sequencing facility. These DNA and RNA are sequenced and converted into data, so called human big data.
This data is used by bioinformaticians, biostatisticians or computational biologists to find the molecular mechanisms behind, like mutation signatures, biomarkers, etc. This information is given back to the physician. It would help the physician to arrive at decisions. This is not a standard protocol procedure in all hospitals, but some hospitals are practicing it now.
What kind of data science methods are you using in your work?
Specifically, we are using a combination of bioinformatics, statistical and deep learning methods for tackling this problem by leveraging the big human data like the data from DNA, RNA and protein levels of the patients. Typically the bioinformatics and statistical methods involve certain processes like alignment, classification, correlation, causal inference and variant analysis. In deep learning, we are developing a series of deep learning models utilizing the sequence-based features and develop a model using neural networks like convolution neural network or Bayesian or autoencoder, whichever would work best for the sequencing data. Eventually the best performing model will be validated against an independent set of tumor genome to determine the performance accuracy or the prediction accuracy. The best results are validated in a wet lab because we have to make sure whether or not the machine is predicting the right thing.
I can imagine that research in cancer genomics is a very vast field. What are some of the challenges involved in research in cancer genomics?
Mainly the genomic data comes with a different file formats because there are multiple genomic data generated using DNA, RNA and all these elements from the human body. They all come in different formats, in varying amounts in terms of their size and there are no uniform APIs. It is really a tedious process to manipulate this data. You can certainly use some Python libraries like NumPy or Pandas for manipulating it to a certain extent. But then there are not enough pythonic ways supporting it currently. Another challenge is to communicate with these complex ideas to the collaborators and group members, since our groups are often comprised of with diverse people from diverse backgrounds. For instance, there are medical doctors, mathematicians and molecular biologists.
So we need to really stay updated in our domain to explain everything a team of people from different backgrounds. For this, I always need to listen to lectures and read papers.
For me it is always a matter of filling my educational gaps. But of course it works well for me, because I can do it at my own pace and timing. However it is a challenge and always I have to stay updated.
And then there are the usual challenges like reducing noise in the data, batch effects and finding the best normalization strategies for the data. Currently we have a collaborator from Heidelberg. He is a wetlab scientist. People who are not working with computer are wetlab scientists. He has a lot of experience in this non-coding RNA field and he is one of our collaborator or talking point where we can ask questions and clarify doubts. It is not a hospital but a group where they do a lot of wetlab experiments to validate the findings from a dry lab, i.e., the computer-based or model-based analysis. So that is a collaboration we have currently.
📬 Get the latest India2Germany articles via email 📨
Cancer, genomics and deep learning are three very hot topics these days. How did you arrive at this topic of research?
As a bioinformatician who was working with human data, most of the tools I was using were based on machine learning methodologies. Deep learning as we all know is a sub-discipline of machine learning. It has also dominated this field recently because it can be used to address variety of questions in the genomics. For instance, understanding how the proteins are binding in the DNA sequences, how certain modifications are happening and predicting expressions of gene from different angles. There are many applications where deep learning can be used.
Despite the number of successes, these deep learning solutions are not adopted very well by the bioinformatics community. Being a bioinformatician myself, I thought of using it. Now the situation is changing. There are a lot of open source tools from big groups like Google Brain out there which one can use. Also we can make small wrappers out of that and use it in our own model. Compared to three years ago presently there are numerous possibilities.
Now is the best time to really use deep learning in genomic research or human data based research
The key to inventing a better drug for cancer is to learn thoroughly from the data from all angles. So deep learning I believe will give us this new look into cancer. We may see things that have been missing for a long time. Thus it will enable an effective diagnosis and accurate diagnosis or bring up some smarter models.
To use deep learning for work is a very important topic of research. So do you know of other groups working in the same area?
There are a lot of other groups; for instance in ETH Zurich, the group where I worked earlier, was the machine learning group. They were using a lot of human data. This was fresh human data coming from hospitals to look into different molecular interactions or molecular mechanisms of patients using these models of deep learning or machine learning models. The other name is Google Brain which is big and well known. At Google, they have a unit called Google Brain and Google Genomics where they are mainly focusing on developing open source tools which can be used by the community later on for free. Then there is a group at Roche (the pharma company) with a small unit where bioinformaticians and machine learning people work together. Also in our group, members of my group are collaborating with Bosch.
These are a couple of examples where people are really working on this topic, but their focus is mainly on image analysis. So they use the images which are coming from the biopsy samples of cancer, e.g., an image of a piece of tumor from breast cancer or kidney. They use those images and try to find biomarkers and help the physicians to make decisions. In our group, most of the people are working on image analysis. They are using images of cancer tumors from brain, breast cancers, and lung cancer. We are another small part of the group working on the genomic sequences.
Being a trained bioinformatician, how did you make the transition to use learning techniques for your work and how did you gain these skills?
Gaining the skills was actually just by working on it, by using the online tools and online resources. I was working with people from multidisciplinary background. Hence it was easy to talk to people and get their help. The groups where I work currently and where I worked earlier were part of universities. So, there are courses available which I can take to learn. We can always do it by our own. These are the ways I learned the skills or continue learning the skills. Also, bioinformatics is data-driven science which uses genomics and utilizes a lot of techniques from machine learning. So I was aware of these techniques. I wasn't using them as a machine learning engineer or a deep learning engineer.
What got you interested in research?
I never planned to be a researcher. I am from India and I grew up in Kerala in south India. I am thankful and blessed to have astonishing parents who give me a lot of freedom. They also had ambitions for me. My mother wanted me to study science and engineering and then pursue Masters and do a PhD in the same field. I did my Bachelor's in Bioinformatics, Master's in Bioinformatics and Systems Biology and my PhD in Bioinformatics. I never changed my field and that was really because my parents had high ambitions for me. But the crucial step happened when I moved to Sweden to pursue my Masters. After completing Bioinformatics engineering in India, I got a chance to pursue masters in Sweden in Chalmers University, which is a technical university. During that time after the first year in the summer break, I got an amazing opportunity to work with a company called Astra Zeneca, where I was working in digitalizing tables and figures from literature about Alzheimer's patients. I put that name on my CV and got to work with small groups in multiple places in Sweden, for instance in Sahlgrenska in Gothenburg and then Scilife lab or Karolinska in Stockholm and so on. Later I got a chance to do my Master thesis with Scilife lab which is like a Hybrid Research Center from KTH and Karolinska. That was the point where I got to work to benchmark some methods which are available for big human data. It was the first time I got exposed to big human data, which was in tera byte size. More than that it was the beginning of cloud computing schedulers, so I got to work with them and with batch systems and sending batch jobs, etc. Honestly this project did a lot for me. First of all it confirmed that I wanted to do PhD. Secondly it was my real foray into learning programming because before that I knew a little bit but not enough. Additionally that was my first experience reading scientific papers, looking into scientific language, how the figures look like, and how things are done. These were very useful for me. I learned how to work independently and to manage data. Most importantly that thesis helped me to decide for PhD. Besides that I loved the autonomy and loved being able to choose the time when I wanted to work. One does not have to work eight hours, one can also work during the night. In research nobody really looks at what, when and where you are working, ultimately the results are important. These opportunities, in fact shaped the researcher in me. By having all these names on my CV it was easy for me to find a PhD. By then I had two published papers in reputed or impactful journals, and it got easier for me to get a PhD position or to be a researcher. So, it happened gradually I would say. The credit largely goes to the mentors and professors I had, and the great colleagues I had. Lot of people contributed to it.
After that I wanted to do PhD in Germany because Germany has got a lot of quality work in cancer research especially at that time. I applied to the German Cancer Research Center (DKFZ) where they were taking many PhD students then and I got this position with Charité and German Cancer Research Institute in 2014. After the completion of my PhD, I got a job in Switzerland as Data Analyst in ETH Zurich. I worked there for one and a half year. Soon after that I got married and due to family reunion I returned to Germany since my husband is from Potsdam. We wanted to live together and we were looking for positions which were nearer to our place. That is how we were looking for Hasso Plattner Institute and I had known Hasso Plattner Institute as my sister who was working for SAP in Heidelberg at that time had told me look into the institute since good work is going on there. I looked into the list of professors in their website, found this professor and I asked him whether or not he could give me a place and thankfully he gave me place and that is how I came here.
In addition to Germany, you have also lived and worked in Sweden, Switzerland and in India. How would you compare your experiences of working in these countries?
To start with a philosophical answer, it ultimately shaped my character or my perception towards the world. First of all I got an opportunity to explore myself and to know myself better. In India I had no idea I would be doing or continuing in research. As I worked with different people I had the opportunities to go to different places and work with different people. I explored that I could do a lot of things and these experiences strengthened me to face the challenges which I didn't have before. In fact we can learn from different people having different work styles. I did not just work with Swedish people only but worked with people from different nationalities, almost from all continents. We can also learn from their work styles.
Some people perfectly plan their time and manage well, some people really know to talk with people and get things done, some people can clearly communicate. So there are different things that we can learn from different people. Eventually we can use all these ideas, and tailor it in a way that will suit own goals and ambitions.
I honestly did that because I didn't have my own pattern, I am sure that there must be people like me. My case was different so I learned from multiple people and I tailored it in a way that suited my goal and my ambitions. If I compare the countries undoubtedly, Germany gives a lot of opportunities currently especially for people with technical skills. One does not need to learn German language, especially people who have computer science skills. Comparing Germany and other countries, cost of living in Germany is really low compared to Sweden and Switzerland. In terms of infrastructure there is not much difference.
What are some of the important topics of research in cancer genomics data?
There are many interesting topics in research. For example, the chances are enormous in immunotherapy, where you can really look into the interplay between the cancer cells and immune system. Secondly in mutational signature, where you can look into why some sort of people who are exposed to different environmental conditions have different sort of mutations and how it is contributing towards cancer. Single Cell Sequencing is another emerging field where you can look into one single cell in a more zoomed fashion and get more ideas how the cells are communicating with one another and how the cell metabolism is happening. So these are some very important topics one can look into.
📬 Get the latest India2Germany articles via email 📨
What would be your suggestions for people looking to work in the area of analysis of cancer genomics data?
If you are looking to work in this field of research, I would really recommend you to stay updated, to read related literature and papers. Now you can even read papers from archives; you do not have to wait until the peer review. There are multiple archives where people are really publishing papers on a daily basis. Use your keywords which you want to look for, then search for the papers, find the paper, read it and try to understand every sentence. If you want to really look for peer reviewed paper, you can use databases like PubMed from NIH and you can use your keywords and find the papers you need and read it. Go through the author names. If you find the paper interesting, then find who is the corresponding author of the paper and write to them and tell them - I like your research. May I work with you? It works, if you are really interested, if you know how to write the mail well, it helps to get some access to the group and can really train yourself with the group. Another thing is networking and collaboration with people. It is also like open talk to people and find what one needs to know. If you are getting any chance to work with the people of your interest or the group of your interest, try getting good reference from the professors and mentors you work with. Go out of the group with a good reference letter.
In Europe, reference letters really help. They go a long way, particularly when working with a good reputed professor.
You can of course use LinkedIn, Google, Glassdoor; there are multiple places you can look for positions. If you want to go into research you can go out. Find the right professor, reading papers you can find the right people and then write to them, go to their web page, write to them and contact them directly. Ask them whether or not they have a position. Always make sure that you have a good CV and a cover letter. Regarding the latter take care and write things like, I like your paper, etc. It should be really good and professional. I really recommend putting a lot of time making good CV and cover letter. I would say, these are the things that really helped me.
📬 Subscribe to our newsletter to stay up-to-date! 👇
India2Germany Newsletter
Join the newsletter to receive the latest updates in your inbox.